
If you need more information about DAGsHub Storage, you can read our Feature Reference # Make sure you are using DVC 1.10 or greater for the next commandĪnd that's it! Just 5 commands and you configured your DVC remote effortlessly, we never opened a cloud provider webpage, handled complicated IAM, or provided credit card information. And finally, push the data to the new remote.
LIST OF USERNAMES AND PASSWORDS DVC HOW TO
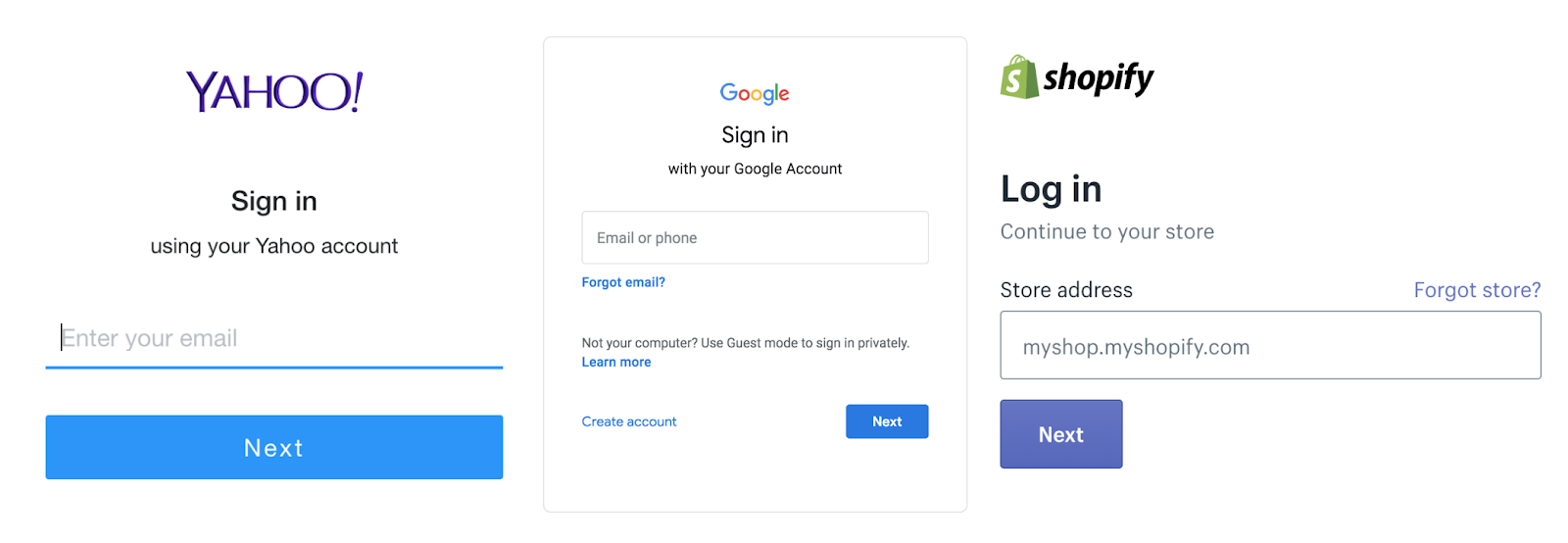
This is the file that will be versioned by Git.įollowing this step we are ready to commit the. In our case DVC created a file called data.dvc, which will look like this outs: gitignore file, so we won't commit it by accident. This command also adds the added entry to the. dvc file this is a small text file containing information about how to access the original entry but not the original entry itself.
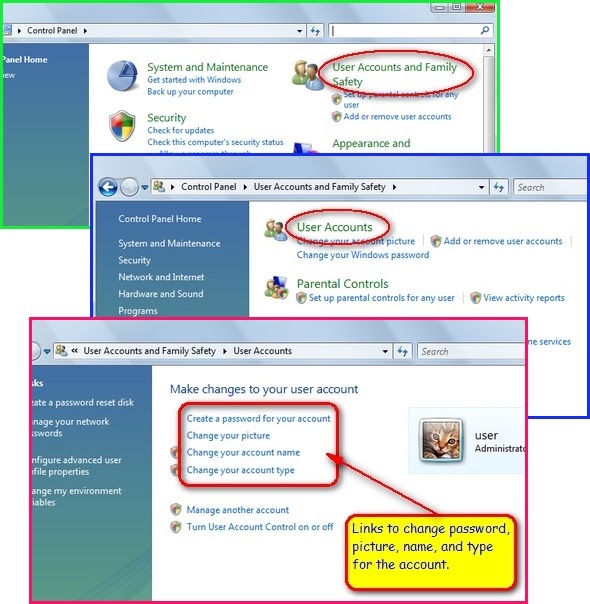
It stores metadata about the entry added in a. To start tracking our data, either a file or a directory, we use dvc add dvc add data These entries can be committed with git commit -m "Initialize DVC"įor the purpose of this tutorial I've created a new project with the following structure data
LIST OF USERNAMES AND PASSWORDS DVC INSTALL
In order to continue with this tutorial you will need to install DVC first.Īfter DVC is installed, in a Git project, initialize it by running dvc init If you need, we have a tutorial on how to start a new project on our platform. There are two ways to do this, either create one from scratch or connect an existing project from any other platform (We support GitHub, GitLab, BitBucket, and any other accessible Git remote). To start, you will need to have a project on DAGsHub.

Just five commands and you are ready to go! To solve this issue, we created DAGsHub Storage, a DVC remote that is super easy to configure, with no credit card, no need to grant complex permissions, and no cloud setup. In this post, I'll show you that this configuration shouldn't have to be so difficult it should be smooth and easy.
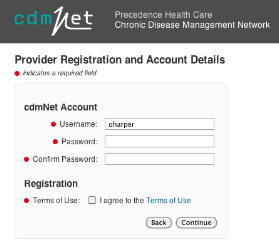
To share your data and models, you will need to configure a DVC remote (such as S3, GCloud Storage, GDrive, etc.), but doing so can be a hassle and take a tremendous amount of time. It also supports pipelines to version control the steps in a typical ML workflow. DVC is a great tool it lets you track and share your data, models, and experiments.
